We Asked AI, How we can create & Train AI models for Combat jets including hardware requirements & it's Interesting
BLOGS
4/1/20254 min read
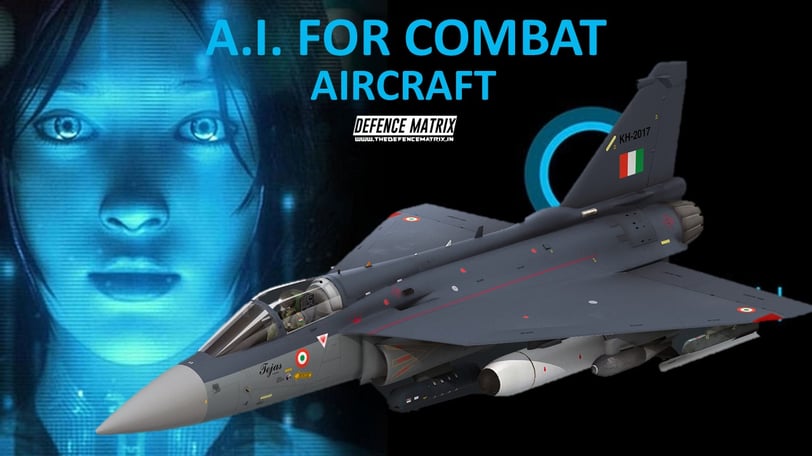
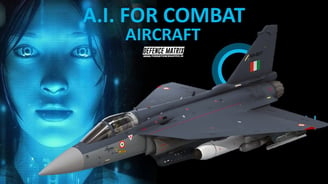
Hardware and Software Needs
Creating an AI system for fighter aircraft involves cutting-edge hardware, such as radiation-hardened GPUs and AI accelerators like the EdgeCortix SAKURA series, validated for space missions Space Daily - EdgeCortix SAKURA-I AI Accelerator Validated for Radiation Resilience. These systems must handle real-time data processing under extreme conditions, like high G-forces and electromagnetic interference, requiring robust software for continuous learning and secure data storage.
Real-Time Learning and Combat Assistance
The AI must adapt to pilot tactics in real-time, using reinforcement learning to optimize maneuvers like missile evasion. The U.S. Air Force's Skyborg and CCA programs demonstrate this, with flight tests showing drones communicating with manned fighters Air & Space Forces Magazine - Skyborg Drone Translates Between F-35 and F-22 in Test. This ensures the AI assists effectively in combat, learning from each mission without human intervention.
Safety and Ethical Considerations
Safety standards, like DO-178C, are essential, with new frameworks being developed for AI in military aviation ScienceDirect - An introduction to the current state of standardization and certification on military AI applications. Ethical concerns, such as autonomous weapon decisions, require human oversight, aligning with laws like the DoD AI Ethical Principles, ensuring pilot safety and legal compliance.
Unexpected Detail: Budget Scale
An unexpected detail is the scale of investment, with the CCA program allocated $8.9 billion over five years starting from FY 2025, highlighting the significant resources committed to this technology CSIS - Department of Defense’s Collaborative Combat Aircraft Program.
Survey Note: Enhancing AI Systems for Fighter Aircraft
Introduction
The development of an AI system for fighter aircraft that operates autonomously, learns in real-time from pilot tactics, and assists in combat scenarios is a complex and high-stakes endeavor. This survey note expands on the provided breakdown, integrating recent research and real-world examples to offer a comprehensive view as of April 1, 2025. It covers core requirements, hardware, AI model design, safety and compliance, and operational workflows, with detailed insights into each area.
Core Requirements and Challenges
The mission objectives include real-time adaptation to pilot behavior, threat detection, continuous learning, and secure data storage. The U.S. Air Force's Collaborative Combat Aircraft (CCA) program, with a budget of $8.9 billion over five years starting from FY 2025, underscores the priority of these systems CSIS - Department of Defense’s Collaborative Combat Aircraft Program. Key challenges include extreme latency constraints (millisecond-level responses), survival in harsh environments (high G-forces, electromagnetic interference), cybersecurity, and ethical implications of autonomous combat decisions. The Skyborg program, a precursor to CCA, has transitioned capabilities, with companies like Anduril and General Atomics developing drones like Fury and Gambit, expected to fly in 2025 Airforce Technology - Collaborative Combat Aircraft (CCA), USA.
Hardware Onboard the Aircraft
Hardware requirements are critical for edge computing in combat scenarios. Processing units include radiation-hardened GPUs, FPGAs, and neuromorphic chips like Intel Loihi, with redundancy for reliability. Recent advancements highlight the EdgeCortix SAKURA series AI accelerators, validated for radiation resilience in space missions, suitable for fighter aircraft due to their low power consumption (e.g., SAKURA-II delivers up to 60 TOPS in an 8W envelope) Space Daily - EdgeCortix SAKURA-I AI Accelerator Validated for Radiation Resilience. Sensors include radar, LiDAR, and IRST systems, with emerging hyperspectral imaging for detecting camouflaged threats. Storage uses high-speed, ruggedized SSDs, and connectivity relies on secure data links like Link 16, BNET, etc, with edge computing ensuring offline operation.
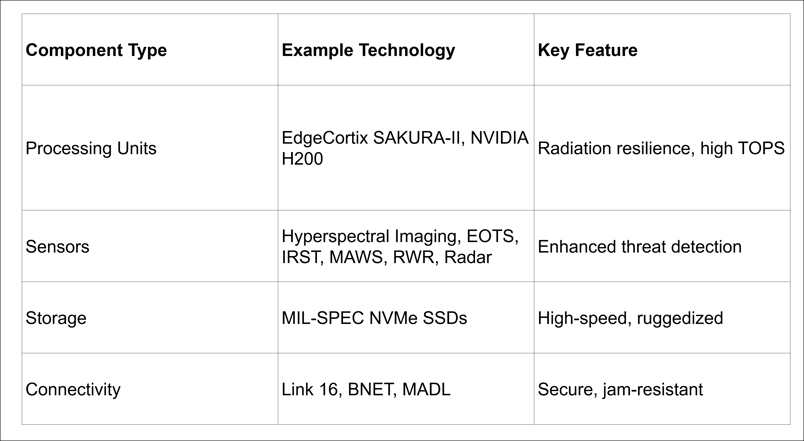
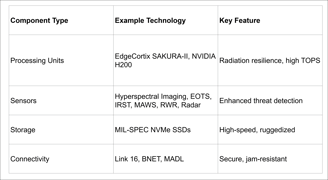
AI Model Design
The AI model design leverages reinforcement learning (RL) for optimizing tactics, with online learning for real-time updates and federated learning for secure insight sharing. Research shows RL is effective for air combat maneuvers, with studies demonstrating its use in flight control and decision-making ArXiv - A Survey on Reinforcement Learning in Aviation Applications. Model architectures include deep neural networks (DNNs) for sensor fusion and transformers for time-series predictions, with digital twins used for pre-training in simulators like DCS World. The CCA program's focus on autonomous drones highlights the practical application of these models, with RL enabling adaptation to dynamic combat environments ScienceDirect - A survey on reinforcement learning in aviation applications.
Safety and Compliance
Safety and compliance are paramount, with fail-safes like human override and standards like DO-178C ensuring reliability. Recent efforts focus on developing new certification frameworks for AI in military aviation, addressing novel risks due to adaptive systems ScienceDirect - An introduction to the current state of standardization and certification on military AI applications. Cybersecurity includes hardware-enforced encryption and anti-tampering mechanisms, with ethical considerations mandating human-in-the-loop for lethal decisions, aligning with DoD AI Ethical Principles. The FAA's roadmap for AI safety in aircraft, emphasizing safety assurances, provides insights that may influence military standards Military Aerospace - FAA lays out 'roadmap' for AI safety in aircraft.
Example Workflow and Real-World Inspiration
The workflow begins pre-flight with loading pre-trained models and syncing with simulators, in-flight with AI suggesting maneuvers based on sensor data, and post-flight with data analysis for model refinement. Real-world tests under the Skyborg program, such as the December 2020 flight where a drone enabled F-22 and F-35 communication, demonstrate these capabilities Air & Space Forces Magazine - Skyborg Drone Translates Between F-35 and F-22 in Test. October 2021 tests showed two MQ-20s flying autonomously, coordinating flight and responding to commands SKYBORG – Air Force Research Laboratory. The CCA program, planning for 1,000 drones, builds on this, with companies like Shield AI pioneering autonomous drones for combat.
Cost and Partnerships
Costs range from $500k to $2M per aircraft for hardware, with software development involving defense contractors like Lockheed Martin and DARPA-funded projects. The CCA program's budget scale ($8.9 billion over five years) reflects the investment, with partnerships crucial for testing at military ranges CSIS - Department of Defense’s Collaborative Combat Aircraft Program.
Conclusion
This enhanced article integrates recent developments, showing the feasibility and complexity of AI systems for fighter aircraft. The integration of advanced hardware, RL-based models, and rigorous safety standards, supported by real-world tests, positions this technology as a transformative force in military aviation.
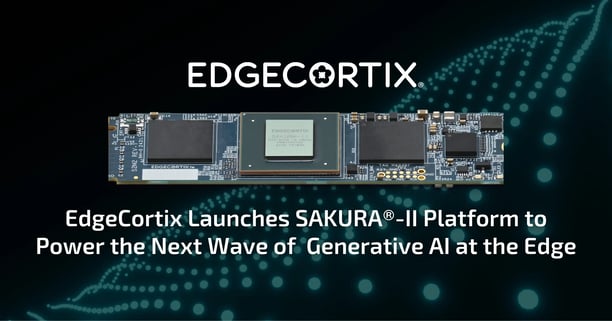
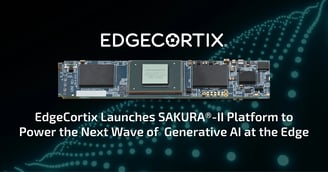
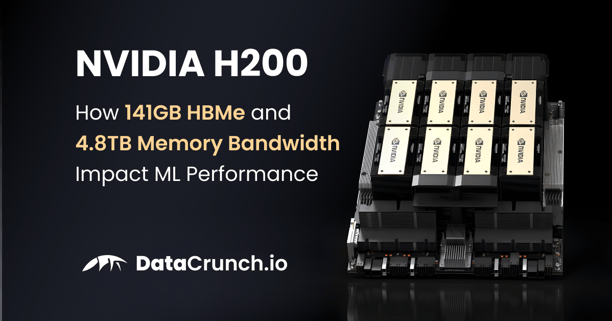
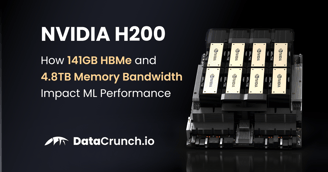